Image Bounding Box and Computer Vision Services
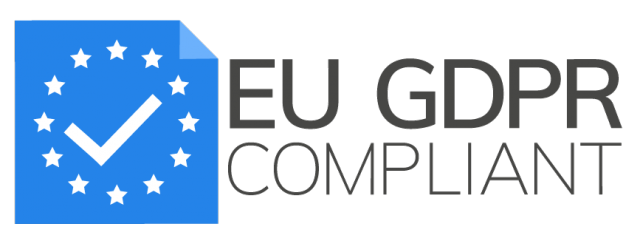
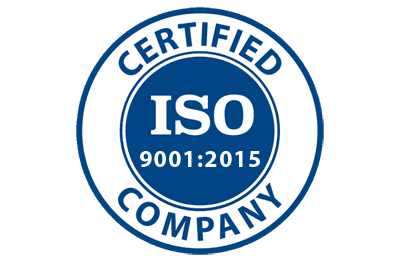
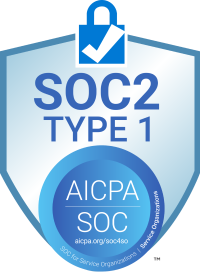
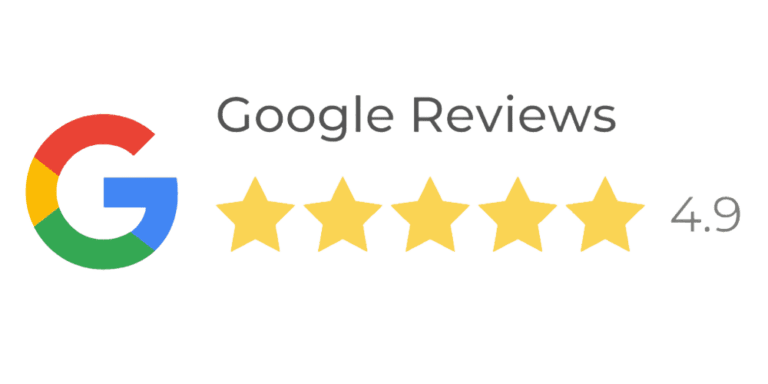
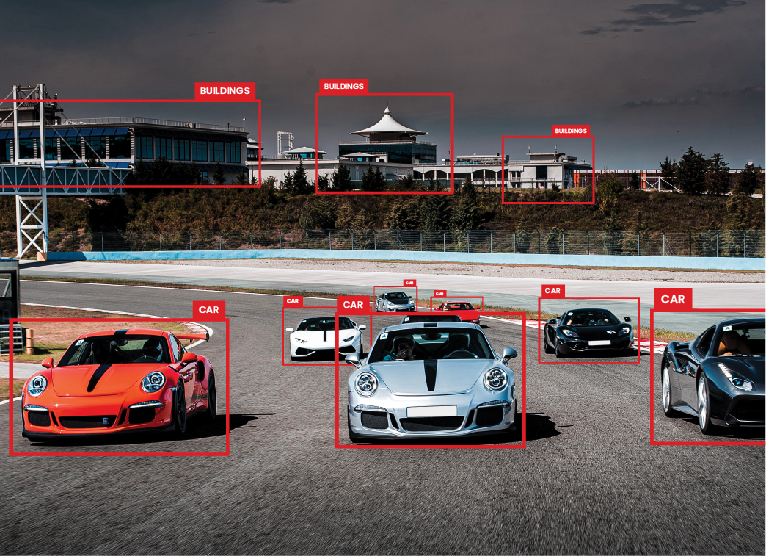
What is a bounding box in image annotation tools?
Image bounding boxes are drawn on objects in an image. Labeling the object of the image makes the thing identifiable to ML & AI Models. Image labeling bounding boxes extract information from the pictures for the artificial intelligence to understand such details.
What are the different types of bounding boxes?
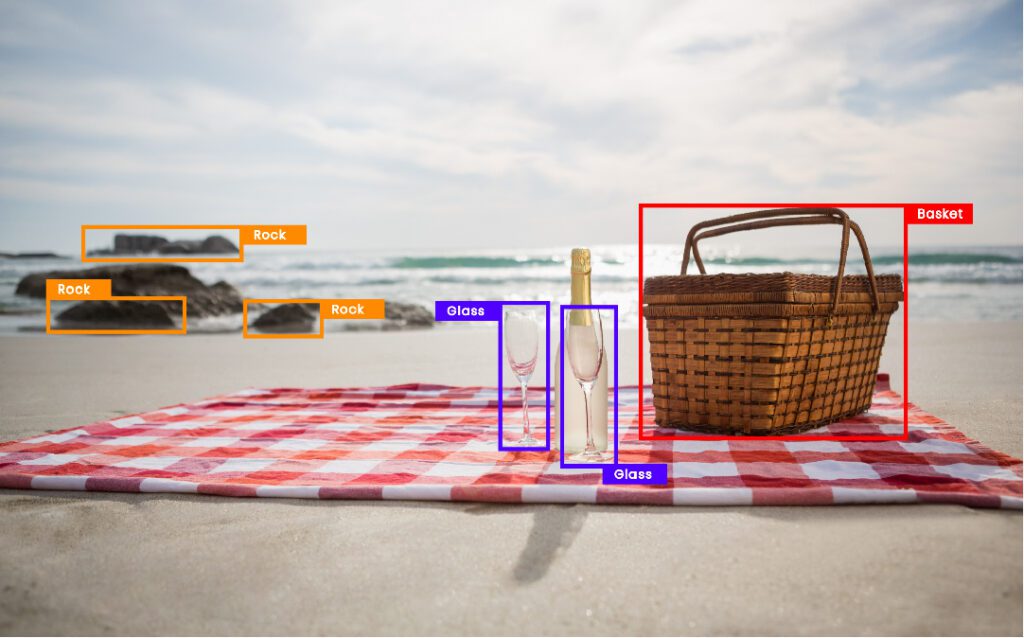
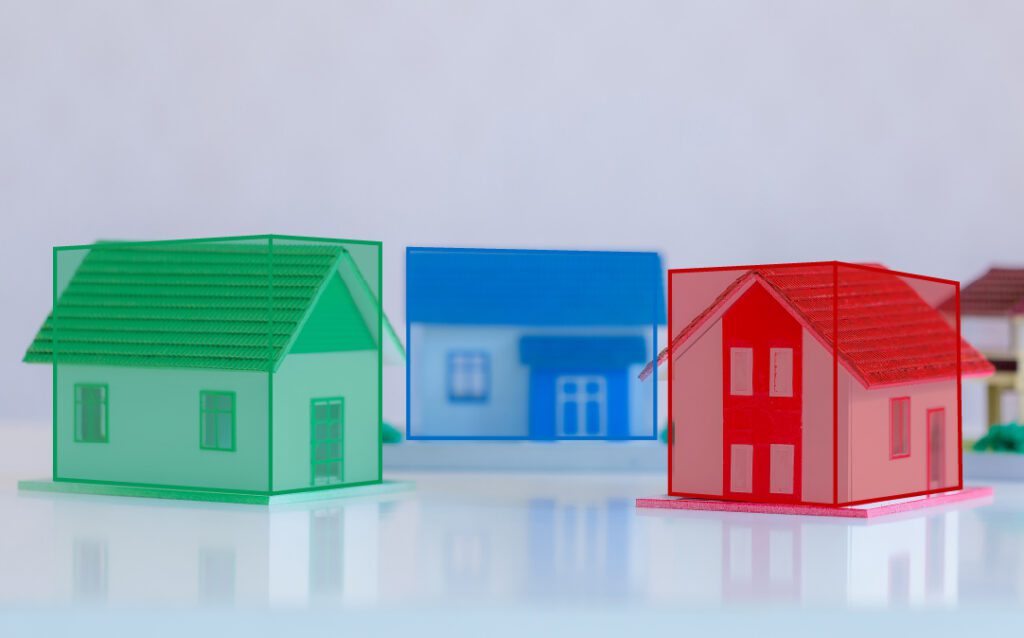
2D bounding box annotation
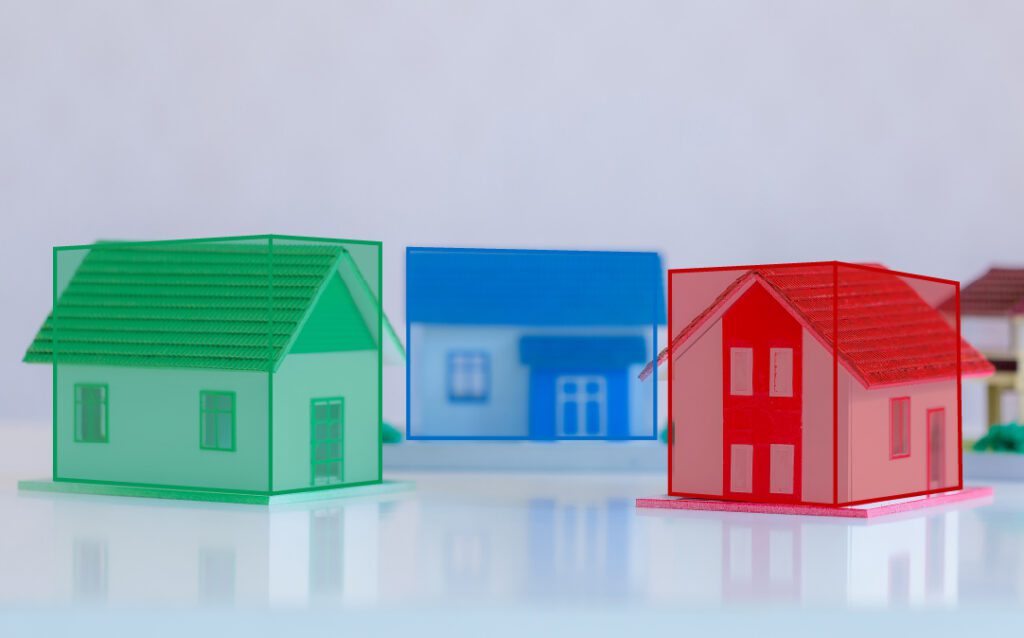
3D bounding box annotation
3D bounding box for higher accuracy
3D bounding boxes annotation carries information about the height, depth, and length of the objects in the images resulting in higher accuracy of image classification than the 2D bounding boxes.
Also, 3D bounding boxes produce more defined edges of the objects in the images compared to 2D Bounding boxes.
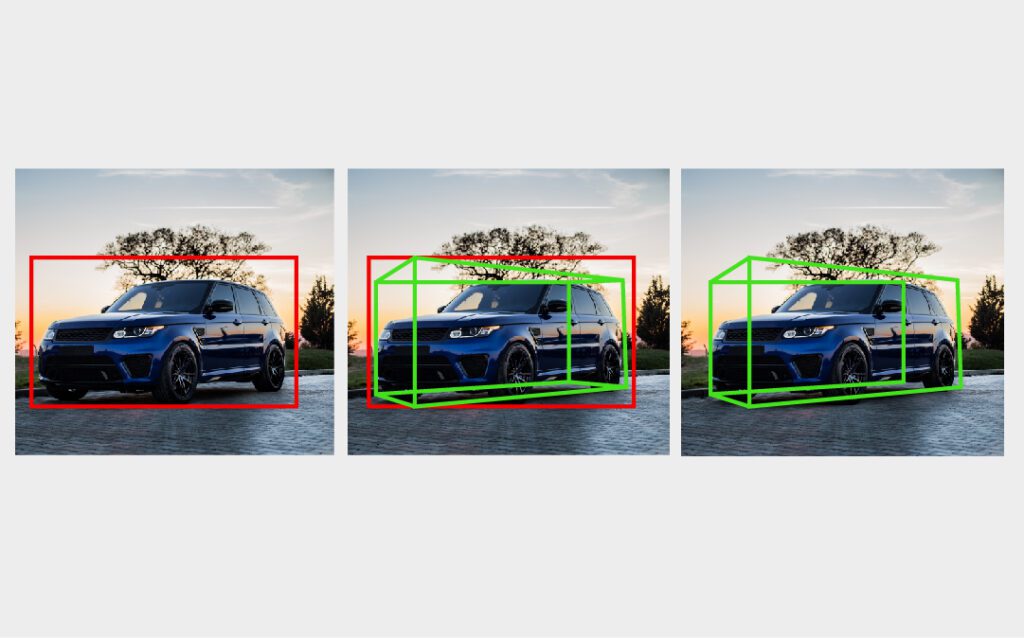
Bounding boxes are used across Industries
Look no further! Let our subject matter experts take care of your data annotation and labelling needs.
Our beliefs behind each annotations

Data Security & Privacy
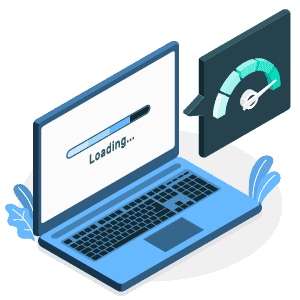
Fast Delivery with High Accuracy
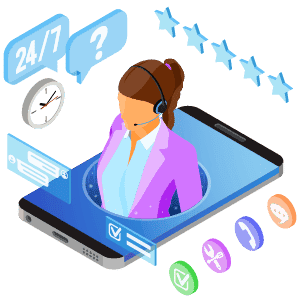
Cost Effective Pricing
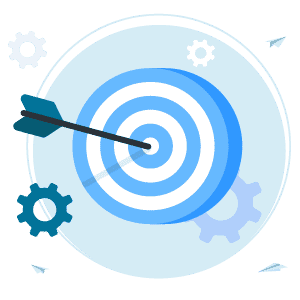
Scalable Solution by Experts
- We are EU-GDPR compliant and SOC 2 Type 1 organization. Data security & privacy is non-negotiable for us
- Our scalable annotation solutions are driven by specialists with years of experience in AI & ML
- We deliver fast and achieve 95%+ accuracy in our annotations
- We aim to achieve highest client satisfaction while providing low cost annotation and fostering longterm relations
Image labeling for deep learning is carried out to ease object detection for a machine. It is done using semantic segmentation, 3D cuboids, and polygon annotation, where every object of interest is labeled and named according to the instructions. The labels must be clear, and it is therefore advised to use the appropriate labeling tools.
The process of labeling data for machine learning is accuracy-driven. Every object of interest in an image is labeled as per the instructions. You can achieve the labels using cuboids, semantic segmentation, or polygons. The labels are then named per the annotation guidelines, enabling easy identification and differentiation by the machine learning model.