Artificial Intelligence has become indispensable in our day-to-day endeavours, from smart locks, conversational AI devices like Google Assistants, driverless vehicles, and automated home systems to intelligent devices. However, all these machines do not just happen to be intelligent. They learn like humans to perform such functions accurately and efficiently in machine learning. The basis behind all such technical jargon is data annotation. Let us break down these highly technical processes to understand everything about data annotation.
Before discussing data annotation, it is essential to get a glimpse of the underlying fields and their inter-relations.
What is Machine Learning?
Machine learning is premised on the idea that machines and programs can perform functions and processes that mimic human cognitive processes without human input. In short, machine learning provides a mechanism through which machines and programs act like human clones where they can make decisions that only humans are thought capable of making. It is intended that these machines can self-learn with machine learning to become better at the tasks they perform. Machine learning seeks to mimic human cognitive functions by utilizing concepts such as deep learning and neural networks to achieve this.
What is Artificial Intelligence?
Artificial Intelligence, or AI, is meant to give machines the experience with world objects so they can learn and gain the ability to distinguish different objects. Similar to how humans acquire intelligence through continuous practice, devices gain intelligence through what is referred to as AI training. In AI, a machine is taught using data to understand different objects’ differences. The more training data an algorithm analyzes, the more intelligent it becomes. Fundamentally, training data is very critical to machine learning and AI algorithms.
The training data must be precise and accurate; otherwise, the resultant model will not know what to do with various objects. That is the role of data annotation. Data annotation provides the data sets needed to train an AI algorithm to perform a particular set of tasks.
What is Data Annotation and Labeling?
As mentioned earlier, machines cannot tell the difference between a cow and a car if left alone. However, machine learning and AI training teach machines how to distinguish the two. The algorithm needs to be fed with data to help it determine the two objects for this to happen, a process known as Computer Vision. That is where data annotation comes in.
Preparing data that is used to train the AI model to understand and classify different objects is called data annotation. It involves tagging, labelling, and attributing additional metadata in a data set so that machines can understand the information.
Why is Data Annotation and Labeling important?
Computers and algorithms have proven they can become a human companion by providing faster, more accurate, and real-time information at rates that human minds cannot. The efficiency with which machines achieve such results has increased interest in having intelligent systems. However, machines cannot learn to do this alone because they lack inherent intelligence. They need to be trained on what to do, making data annotation very important.
AI models are developed by continually providing them with vast training data to analyze and deliver outputs. The training data, created through data annotation, helps speech, computer vision, and recognition models identify elements in real-world applications and produce relevant and accurate output. However, without data annotation, machines would remain machines.
Given that each ML & AI models has a different end goal, the training and data curation processes are also customized basis the needs of the modules. The different types of annotation (image annotation, video data labeling, text annotation and audio annotation) and the nitty-gritty behind such annotation tools are equally important to understand.

What is the difference between Data Labeling and Data Annotation?
The distinction between data labelling and data annotation is very blurred. Often people use these terms interchangeably to mean the same thing. However, there are several differences between the two. For data annotation, AI models label relevant data to make it recognizable. Data annotation is the basic foundation of machine learning.
Data labelling involves adding metadata to a set of data to allow the training of ML models. Data labeling helps ML models identify relevant aspects of a data set.
How much training data is required to train AI & ML algorithms?
As in humans, learning is a continuous process and never stops. Machines need to learn to become better at what they constantly do. The more training data they process, the more accurate results will become. There is no generally agreed limit regarding the volume of data that should be used in training a model.
Similar to human learning, some subjects require more learning than others based on the complexities involved. It is similar to machine learning, where even the simplest tasks need constant training to achieve accurate results. Without continuous movement, the AI model would misidentify objects, giving inaccurate results.
How to get free training datasets?
Getting annotated and labeled data is the largest pain point for many data scientists. Getting training data can be daunting if the AI & ML algorithms’ end goal is unclear. Also, because of the variability of goals, obtaining trained data to match the needs of the algorithms can be difficult. Hence, companies usually get the raw data annotated and labelled to prepare the training data basis the requirements and needs of the AI & ML Algorithms.
Some of the sources where raw data and trained data sets are available are mentioned below:
Open source datasets for training
There are accessible avenues to get massive volumes of data that a data annotation project can use. The advantage of such sources is that the data is out there freely. Some examples of such free sites include:
- Forums such as Reddit & GitHub can quickly obtain vast amounts of data. If data is unavailable, these forums are resourceful as one can reach out if in need of particular data sets.
- Google: In 2020, more than 240 million datasets were released by Google. These datasets are readily available and for free.
- Kaggle: It is a free site to obtain data sets besides the ML resources it provides.
Data Scraping
Data scraping is an alternative to obtaining training data, especially when there are no freely available data sets. Data scraping involves getting data from different sources such as journals, profiles, and public portals in data mining. However, specialized tools are needed to scrape data. Also, it is essential to overcome the legal challenges around copyright and usage issues.
Outsourcing
When it is impossible to find free datasets and data scraping is not an option, the third option is outsourcing the process of obtaining data to external vendors. Outsourcing is a deal as it removes the responsibility of finding the correct raw data for the AI & ML algorithms, and resources can be diverted into building the model. The data annotation vendors carry out all the tasks from data scraping, cleaning, and classifying, making the process seamless, easy, and efficient.
How to get Data Labelled and Annotated?
Data annotation and labelling require advanced platforms and tools to accurately and securely prepare raw data to become training data. Managing and working on such tools, for example video bounding box annotation tools, require a highly skilled workforce and data annotation specialist. For such reasons, most organizations outsource their annotation and labelling needs and leave it to experts who achieve higher efficiency and lower overall costs.
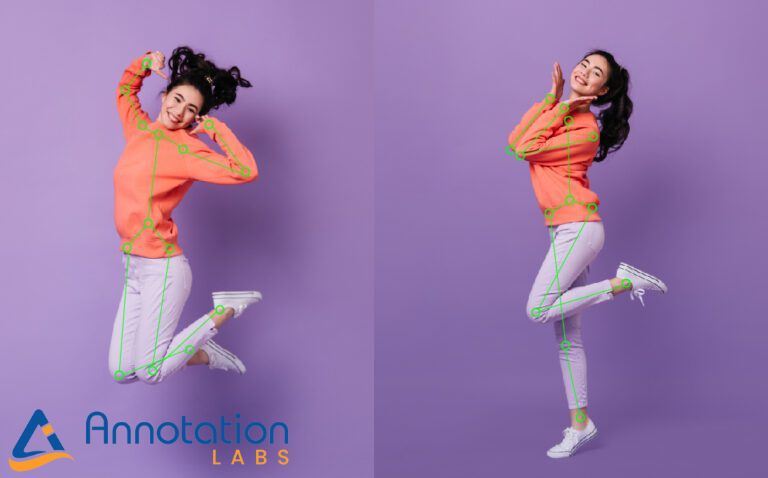
Those who cannot afford to outsource or build their custom annotation tools can turn to some of the open-source tools readily available in the market. Most of these free tools are designed to deal with specific data types, which can be a limitation when training modules with multiple data types.
How to chose correct Data Annotation Tools and Processes?
Selecting data annotation tools seems simple, but there are complex intricacies to consider before deciding on one tool. One key consideration is the use case. The tools selected must align with the annotated data’s intended use case. It is also essential to consider personal requirements such as network, cloud, or local and tools’ availability per the annotation requirements.
Data annotation experts guide the best and most efficient method of preparing training data with their years of subject matter knowledge.
Quality is also an important aspect. One must consider the quality of output obtained when using the tool and how that affects the quality of the AI model. The critical question should be whether the selected tool can annotate the data accurately so that the model will produce effectual output once it is trained.
Understand your Annotation needs better
This guide provides a comprehensive explanation of data annotation. It explains factors that necessitate data annotation, how it works and other underlying factors. It closes by offering insight to anyone interested in carrying out data annotation services on what they should consider when deciding on the platform to ensure they end up with an AI model churning out garbage.