The last century has seen tremendous advancement in healthcare technology. However, in the 21st century, technology seems to be taking the health industry by storm. Technological advances in machine learning and AI have revolutionized how we access, experience, and interact with medicine and significantly impact our view of the medical and healthcare industry.
Nowadays, the most consequential human health conditions are being handled through technology. A new field called AI Healthcare has developed within the broader field of AI, focusing on advancing ML and AI for Healthcare and Medicine. Currently, ML models are being used to predict the onset of severe medical conditions such as cancerous tumors and hereditary diseases. Using medical computer vision models, surgical procedures are being optimized using AI, where robot surgeons and intelligent surgical tools are used to reduce after-surgery recovery times. Every day, dreamers are innovating ways to deliver healthcare better using ML and AI.
How is big data and data analytics relevant in Healthcare?
Before delving into the roles ML and AI are playing in the healthcare industry, it is essential to understand how big data analytics in the healthcare industry assist in achieving these technological advancements. It has become easy to access massive amounts of data about human health conditions and activities in recent years. The data is collected from different sources, including digital platforms, patients’ electronic health records, wearable devices, analysis of medical images, and gene sequencing. The resulting massive amounts of data result in what is called big data and thus need efficient methods for data extraction in healthcare.
Big data is playing a critical role in the growth of ML and Healthcare AI. Such vast amounts of varied data allow scientists to analyze crucial information that gives insight into how people’s health is affected by certain lifestyles, what medical conditions are prevalent, and their probable causes. In healthcare AI, big data is crucial as it provides ready healthcare datasets, such as images of healthcare conditions or medical text classification dataset, that can be used to train the models to produce accurate results. Even the most straightforward medical applications available online rely on big data to train the models. From the vast sets of data available in big data, healthcare professionals and experts annotate every element relevant to the AI model. Without such a rigorous annotation process, machines would not know what to do with medical data sets. These annotations include labeling medical imaging data, NLP in medical coding and AI medical transcription, and text analysis on medical text dataset for healthcare chatbots.
Not just healthcare, but read all about advanced data analytics practices in other industries from our research team
What are some benefits of AI in the Healthcare and Medical Industry?
Let us look at some of the benefits of ML, AI & big data in the healthcare industry
Robotic surgery
Surgeries remain an extremely complicated endeavor in human health. Although the risks associated with surgeries have reduced significantly, cases of misdiagnosis and unnecessary surgeries persist. Besides such cases, some surgical procedures are highly complex, putting surgeons under pressure. In the past years, the development of AI-enabled robots has come as a reprieve in the surgical theaters. These robots come equipped with enhanced natural stereo visualization and augmented reality. They can understand scenarios and respond appropriately during surgical procedures. To achieve precision, surgery robots need AI-enabled robots have also become an essential part of the surgical decision-making process. By getting trained on medical data sets, these machines can determine whether the patient requires surgery or the current condition can be cured through medication or by prescribing changes in the patient’s lifestyle.
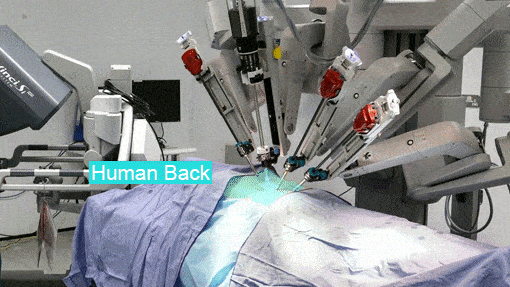
Predicting autoimmune and neurological diseases
Researchers globally have grappled with the question of whether some diseases can be detected early before they reach severe stages. Some of these medical conditions are considered dangerous as they have no cure, nor can they be reversed even with medical attention. As such, focus has shifted to predicting the medical conditions in advance to manage them. AI and ML models have been trained and used to predict cases of such diseases, including dementia, Alzheimer’s, and Parkinson’s. When such conditions are detected early, they can be managed so that they don’t advance to impede a person’s health.
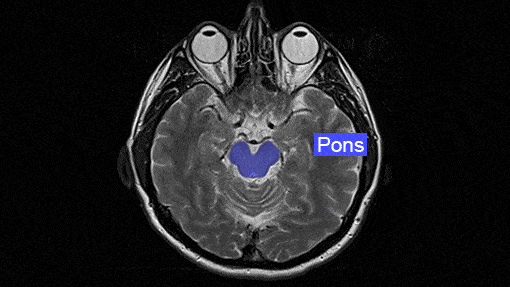
Preparing personalized patient treatment plans
As mentioned earlier, big data has become an essential aspect of healthcare. With access to volumes of medical datasets, AI models are being trained to analyze patient data and prepare treatment plans fitting their condition. Such AI-driven treatments rely on patient health history, robustness of AI medical diagnosis engines, and history of allergies to recommend effective treatment plans. This increases the rate of recovery and reduces cases of admissions and readmissions
Virtual patient assistants and nurses
During the pandemic, one lesson learned is that our health system is always a pandemic from being overwhelmed. This is mainly for medical workers who are stretched thin and work round the clock during such times. Advancements in healthcare AI provide hope by promising to have virtual nurses and medical chatbot assistants who can take care of patients and help their recovery journey. The virtual digital nurses are trained to carry out all duties and activities of a human nurse. It is also expected that the digital nurses will be instrumental, especially when dealing with outbreaks of highly transmissible diseases where they can be used to reduce human contact to safeguard the healthcare workers.
Disease and pathogen profiling
Diseases can be hazardous if there is no scientific knowledge about the pathogen causing them or how they are spread. In such cases, scientists have to walk blindly as they try to sequence the genome of the pathogens, which exposes them to significant risks. With AI models, these risks are minimized as viral diseases can have their pathogens genomically sequenced and profiled safely.
Telepresence physicians
Research into telemedicine has picked pace in recent years, and such technology is already in use in some places. Telemedicine involves examining and treating patients remotely without having the necessary physician physically with the patient. Telemedicine allows patients in rural areas to access quality and affordable healthcare without traveling to urban centers. Telepresence physicians are AI-powered robots that can diagnose and provide a treatment plan for patients while creating an atmosphere resembling a regular patient-doctor interaction.
These robots are designed to answer patient questions, ask questions, and diagnose the patient’s condition based on the pre-annotated data. In the event of critical situations, the robots are capable of seeking further human intervention. Through the AI cameras, the robots can provide a realistic patient assessment and mimic the human doctor’s diagnosis process.
Prescription dispensing
Dispensing prescriptions for patients is usually a repetitive process requiring accuracy. AI robots have been developed to speed up the process and increase accuracy. These robots reduce the risks of inaccurate doses and increase efficiency in terms of timely patient treatment.
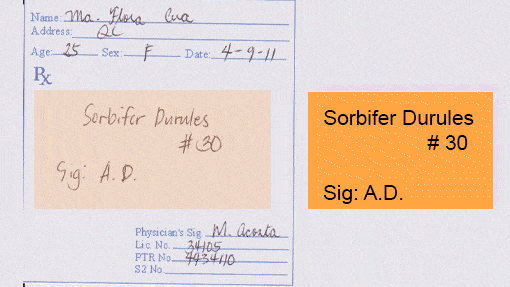
There are other numerous areas of application of AI and ML in healthcare. In all these applications, the common denominator is that AI robots increase the accuracy, efficiency, and speed of medical conditions identified, diagnosed, and formulated treatment plans.
What are the challenges in developing AI solutions in the Healthcare Industry?
The development of healthcare AI solutions is not without its own set of problems. As researchers and innovators bury their heads in trying to advance AI technology in healthcare, here are some of the challenges they face:
Data constraints
As indicated earlier, healthcare AI is data-driven. There should be a constant generation of healthcare data to train the ML models. For example, training models for Chatbots need a large amount of medical dataset for text classification. Training robots for surgery needs highly accurate medical image dataset for training of the AI algorithms. However, such data is not available for one reason or another. Data collection in healthcare is a universal issue thanks to the non-uniformity of data collection standards in the healthcare industry. In such cases, it means that the development of the AI models has to be put on hold until such data is available. This could slow down or make it impossible to develop healthcare AI models.
Legal and ethical issues
Healthcare is among the most regulated fields. Patient data is regarded as private, and obtaining such data takes time and requires numerous legal approval. Further, multiple protocols and standards dictate what can and cannot be done with patient data. It, therefore, becomes tedious as organizations are often needed to do data de-identification, follow stringent rules and standards, and deal with interoperability of data requirements. Data sharing is fair among all stakeholders.
Constrained number of professional medical data annotators
As mentioned, one goal of having healthcare AI is to increase the accuracy of the results. This means that the model training data must be accurately annotated and labelled datasets to achieve the desired accurate results. However, the challenges arise from healthcare being a highly specialized field. The specialization makes it challenging to recruit data annotators for annotation tasks. This can be a massive challenge as, without accurately annotated data, models cannot be trained to perform their intended functions. One of the pioneers of the industry is Annotation Labs with their expert annotation and labeling solutions for Healthcare and Medical Industry.
Read more about their advanced work methodology and security standards they follow.
What is the future of big data, and machine learning in the Healthcare space?
AI advancements are being witnessed in all sectors. It is expected that going forward, further advances in health technology will play a significant role in growing healthcare AI. The need for faster, efficient, and timely access to healthcare is expected to continue to drive more innovations in healthcare AI. Besides, governments worldwide are gearing to improve the healthcare systems of their populace, and one way to do that is through ML/AI. However, it is essential to note that a key to achieving our goals is by leveraging the power of big data.