The future we have always thought about, where machines and humans interact in a symbiotic manner, is here with us. The journey to achieve this feat has come a long way thanks to the power of computer vision and data annotation. Back then, communication was one way, with humans talking to machines. Such communication has dramatically changed as engines can now analyze and process our facial appearances to determine our mood in real-time. The advancement in this area is all thanks to the field of computer vision.
What is computer vision?
Computer vision allows computers to detect and understand real-world elements through cameras with the help of artificial intelligence and machine learning. Computer vision will enable machines to detect and identify different objects, including details such as make, model, manufacturer, and age. It also allows people to scan documents and convert them automatically to their preferred format or scan questions and have answers automatically. Computer vision goes far beyond what we can imagine. Another form of computer vision is AI image search which is being implemented in social media sites where it is possible to detect faces upon uploading an image.
How does computer vision work?
Computer vision is a broad field within which other subfields are crucial to computer vision development and technological achievements. It is important to note that, as most people understand, computers and machines are generally dumb. They cannot recognize an object if they are not taught how to. That makes training of these machines essential to achieve image recognition which is the fundamental technology behind computer vision.
Image recognition and object detection, as mentioned, is the fundamental element of computer vision that allows machines to detect, analyze and identify objects in the natural environment. Through image recognition, machines can mimic the human brain, where they detect, analyze, and draw informed conclusions from objects, also known as image processing using machine learning. Unlike computer vision which is broad object detection, image recognition and image visual search specifically deal with pixel-by-pixel analyses of objects similar to the human brain.
What is the definition and meaning of image annotation?
So far, we have mentioned computer vision, image recognition, and object detection. Let us now introduce the concept of image annotation. Image annotation or image labeling is the building block for both computer vision and image recognition. For any machine to detect and analyze an object, it has been trained to know how to perceive objects in the real world. The training of such algorithm models running these machines is done using training image datasets that have to be annotated to help the machines understand what to do with various objects.
Therefore, image annotation is a fundamental process in computer vision and image recognition as it is the technology behind the ability of machines to make ideal and intelligent decisions.
How does image annotation work?
Machines cannot distinguish between a cow, a car, and a human. They have to be rigorously trained to identify and distinguish between these objects whenever they interact. Machines need to learn how each thing looks and what attributes and features differentiate it from other objects.
Best way to annotate images or label images involve a specialist image annotator tagging, labeling, and transcribing high quality image dataset to make it possible for AI & ML enabled machines to understand better and identify objects. Image annotation allows machines to learn what, for instance, a cat is. For a device to correctly identify a cat, you need to annotate images of cats and train the algorithm. It would be best if you also annotated pictures that show what is not a cat so that machines do not perceive dogs as cats. Using large volumes of annotated image data, the machine learns to identify each object in the environment seamlessly and accurately.
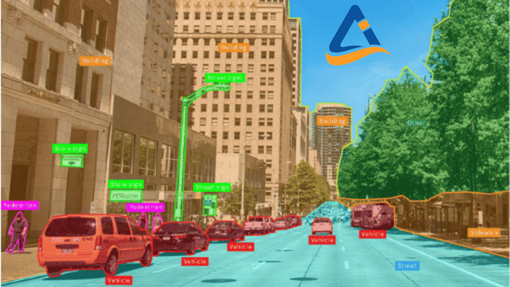
What are the types of image datasets?
Now that we have learned what image annotation is, let us understand the different types of image annotation. Images contain numerous elements, some of which are useful and some not, depending on the intended use case. You can either retain these elements or eliminate them when annotating an image. As mentioned, the decision to maintain or eliminate elements in an image is based on the project being undertaken.
It is important to note that the image annotation process can annotate different types of images for a project. Let us look at each of them individually.
2D images and videos
These are data collection images or videos containing only two dimensions. They include images or videos captured by cameras, SLRs, or optical microscope images.
3D images and videos
These data collection images or videos provide more detailed information about an object as they are three-dimensional. They include images from scanning probe microscopes and electron cameras and LiDARs from LiDAR Cameras.
What are the types of image annotation methods?
Annotating an image can mean different things depending on the type of information and detail captured. In some instances, it could be as easy as classifying the images into other groups, or it could be complex to add details about specific elements of the picture. Let us look at the different types of annotation that can be conducted on an image.
Image classification
Image classification is the simplest form of image annotation. It involves classifying or grouping different objects in an image into specified categories. The process is simple, applying just the identification of objects such as buildings, vehicles, and humans.
Object detection
Object detection builds on image classification. It involves adding more details to objects. For instance, objects classified as vehicles can be cars, taxis, or buses. Object detection provides further information about a particular object or set of objects.
Image segmentation
Image segmentation provides more specific information about an image to achieve a better degree of granularity. Image segmentation involves providing information such as the object’s shape, color, and appearance, allowing the machines to understand each object. Objects are tracked using an outlining tool, and the resulting figure is assigned to a specific class. Segmentation is time-consuming but advantageous compared to other annotation types due to its level of detail specially needed for image segmentation deep learning.

Instance segmentation
Instance segmentation builds on semantic segmentation by providing further information about each instance of a particular object. It provides instance segmentation of an object. For example, two humans are the same object but based on instance segmentation; these are two distinct instances of an object. These will be segmented and labeled as ‘person 1’ and ‘person 2’ in such a case. This added level of granularity allows machines to distinguish between different instances of the same object.
Object tracking
Object tracking is a form of image annotation used for multi-frame images and videos. It involves following an object across several frames and identifying the required elements. It is used to annotate surveillance camera footage and other moving images.
What are the types of image annotation tools?
There are different processes and techniques available to annotate an image. Best image annotation services and companies achieve higher the accuracy with manual image annotations which results in better training of the computer vision models. With their subject matter experts and methodical approach to complex image annotation use cases, they achieve faster turnaround while keeping the annotation costs low. Here are some image classification labeling tools to annotate images:
Image bounding box
The use of bounding boxes is the most basic image annotation technique. Bounding boxes can either be 2D, providing the length and width of the object, or 3D, also known as cuboids that give the object’s depth. For 2D annotation, an annotator draws a tight box around an object. Usually, annotators mark boxes around parts of the thing they can see. However, for 3D image annotation, annotators are needed to approximate an object’s details that are not visible.
Polygon annotation
Polygon annotation is deployed when images to be annotated are asymmetrical and such details need to be captured. It involves placing dots along the boundaries of objects and then joining them using lines to join the dots to produce an almost exact outline of the thing. Polygon annotation is essential when the shape is crucial in training the AI model.
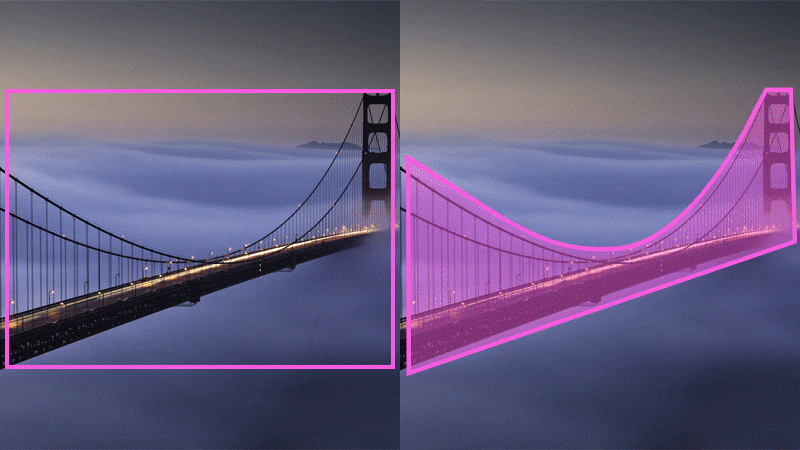
Line annotation
Lines are used as a technique for annotating images. Machines need to understand the boundaries between objects, and line annotation is used. Line annotation is mainly used in autonomous driving, where cars are taught the boundaries between lanes.
Other tools such as semantic segmentation of images, and landmarks and points are also actively used to annotate images.
What are the applications of image annotation?
There are numerous cases where image annotation plays a critical role. Let us look at some of image annotation applications briefly:
- In retail and shopping where images are annotated to identify different products
- Healthcare where ML models are trained to identify medical image diagnostics and anomalies in human organs on medical reports
- In autonomous cars where cars are taught to drive themselves using car image recognition models by identifying different objects and making necessary decisions
- Face detection uses AI algorithms to detect human faces, even picking them from a crowd.
- Emotion detection: A breakthrough in image recognition allows machines to detect human sentiments such as sad, happy, or neutral, thus enabling product sentiment analysis.\
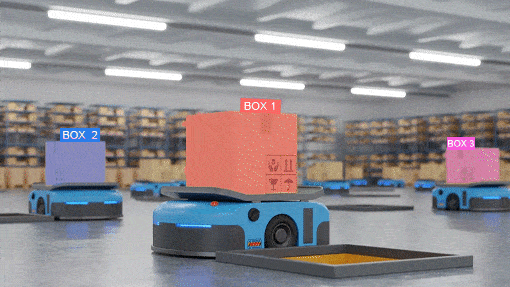
Future of image annotation services?
Image recognition is rapidly growing, and more advancements in this field are expected in the future. With broader use and adoption, the need for image annotation will continue to grow. For anyone wanting to become an image annotator, that is to say, workflow for such tasks will grow continually in a future where AI, particularly computer vision, is promising to be everywhere.